

On the horizon: Precision disease management for Crohn’s disease
U-M team developing image analysis tools to offer individualized treatment assessments for a painful and burdensome chronic condition
Crohn’s Disease (CD), which is estimated to affect nearly 1 million people in North America, can cause a range of debilitating symptoms, including abdominal pain, severe diarrhea, fatigue, weight loss, and potentially permanent structural damage to the intestine.
With onset typically between the late teens and late 30s, this inflammatory bowel disease can seriously hamper quality of life during the years normally vibrant with activity, discovery, and personal growth.
Effective treatments for the intestinal inflammation at the root of these symptoms are widely available to provide some relief and prevent serious complications. However, as with a number of other chronic conditions, it is difficult to predict who will benefit most, or at all, from these treatments.
Determining the value of each treatment for each individual is critical, as these immunosuppressant and biologic therapies carry significant risks of potentially serious side effects, and can cost between $15,000-$100,000 a year per person. For young people who will need to manage the disease for the rest of their lives, this can be an incredibly expensive proposition.
Experienced physicians integrate clinical, laboratory, and imaging data, as well as the patient’s particular needs to select a therapy. For less uncommon conditions like Crohn’s disease, however, expert providers may be a great distance from the patient. Even with access to an expert, detailed data analysis can be impractical within the constraints of a typical doctor’s visit.
Now, a team of University of Michigan researchers led by Ryan Stidham, M.D., M.Sc., a University of Michigan gastroenterologist, is developing a computer-based tool that could rapidly assess CT scan images, laboratory data, and prior treatment history to help provide a more customized individual treatment plan for anyone with a Crohn’s diagnosis, even those living at a great distance from specialty care, and at low cost.
The team is using machine learning and custom image recognition software developed at U-M to train computers to assess Crohn’s disease like a seasoned expert physician. While machine learning is slowly becoming a routine analytic method, the ability train machines to understand the medical relevance of organ features on CT scans has never been performed in Crohn’s.
Challenges in diagnosis and access
Currently, CD is evaluated as mild, moderate, or severe, based on an individual’s symptoms, and these criteria are used to make decisions about the type and strength of treatment. Yet two people labeled with the same symptom severity can end up with extraordinarily different outcomes. It turns out that these broad descriptors are not very specific to individuals’ unique characteristics and other problems that determine the course of their disease.
Imaging data of the digestive tract from CTs and MRIs, in conjunction with laboratory testing and colonoscopy, can provide the missing components of a more richly detailed portrait of a person’s individual Crohn’s disease features – describing exactly where the disease is located along the intestine, and distinguishing varying degrees of damage from one part to another. Imaging features, such as intestinal thickness, blood flow, and length of involvement can tell much more about a patients Crohn’s disease than symptoms, labs, or even colonoscopy.
This imaging information is highly predictive of whether an individual will respond to a medication or not, and their likelihood of developing future complications. Such predictions could make the difference between knowing whether changing medical therapy type or intensity is likely to help an individual with Crohn’s improve and avoid future complications, whether medications are likely to be insufficient and the patient should see a surgeon, or whether simply treating symptoms with a short-term therapy, sparing long-term suppression of the immune system, would be best.
However, extracting the relevant information from these imaging studies to make these predictions typically requires a radiologist with substantial experience evaluating CD. And even when an experienced radiologist is available, the detailed measurements needed can be very time consuming, difficult to quantify, and may vary depending on which radiologist interprets the study.
This is where Stidham and team’s project comes in.
How it would work
The tool in development can import imaging studies, including abdominal CT and CT-enterography studies, and then identify the major layers of the intestine where disease can occur. Machine learning methods, which can train software to efficiently extract precise measurements of the bowel’s features, similar to information a radiologist would collect.
“This will offload an often tedious task from radiologists, giving them more time and data to make an interpretation,” Stidham explains. “Our goal is to provide a preliminary automatic assessment of Crohn’s activity that can provide a rapid prediction for patients and their doctors,” he adds.
As the model is refined and implemented in real-world settings, it would continually improve its functionality by learning from the combined imaging, treatment, symptom, and patient outcome data and refining its predictive abilities based on that previous experience.
Broadening access to a scarce resource
“This approach offers a number of advantages: rapid analysis, minimal variation in measurements, and improved access – the machines needed to do this could run 24-7 at very low cost,” Stidham says. “Plus, the patient doesn’t have to be near a center of excellence to have access to assessments and predictions from this type of technology.”
For the individual with Crohn’s who may present to their local physician or an emergency room that may have limited experience with CD and may be weighing additional therapy against immediate surgery, for example, “this kind of tool could really help acute decision making when experts are not immediately available,” Stidham says.
Ultimately, the team is working towards developing the capability for a clinician anywhere to be able to forward a patients’ CT scan to their server, have it analyzed for Crohn’s features, and quickly receive a report for individualized treatment guidance.
“The information needed to make big leaps in our ability to individualize care are available from existing diagnostic tests – it’s just a problem of efficiently extracting that data,” Stidham explains. “And that’s what our research is about – making tools to extract granular data that give a clearer picture of an individual’s disease.”
"I think this will provide the building blocks to improve the way we interpret results and deliver care for Crohn’s Disease,” Stidham says. “We believe the development of these risk prediction tools will overcome some of the most critical obstacles in Crohn’s disease management to deliver the right treatment, at the right time, for the right patient.”
This work is supported through grants from the Department of Defense Peer Reviewed Medical Research Program and a K23 career development award from the National Institutes of Health. Stidham’s collaborators include Binu Enchakalody, Akbar Waljee, Mahmoud Al-Hawary, Ashish Wasnik, and others in the Morphomics Analysis Group at the University of Michigan. Stidham and Waljee are members of the Institute for Healthcare Policy & Innovation.

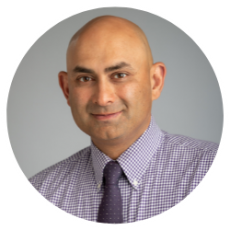